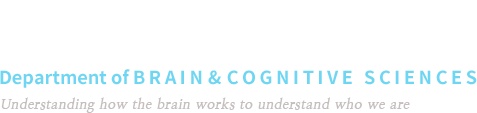
Faculty


Faculty Publications
Postoperative seizure outcome guided machine learning for interictal electrocorticography in neocortical epilepsy. | |
---|---|
Year of publication | 2018 |
Title of paper | Postoperative seizure outcome guided machine learning for interictal electrocorticography in neocortical epilepsy. |
Author | Park SC,Chun Kee Chung, |
Publication in journal | Journal of neurophysiology. |
Status of publication | accepted |
Vol | 119 |
Link | http://doi.org/10.1152/jn.00225.2017 246회 연결 |
Abstract The objective of this study was to introduce a new machine learning guided by outcome of resective epilepsy surgery defined as the presence/absence of seizures to improve data mining for interictal pathological activities in neocortical epilepsy. Electrocorticographies for 39 patients with medically intractable neocortical epilepsy were analyzed. We separately analyzed 38 frequencies from 0.9 to 800 Hz including both high-frequency activities and low-frequency activities to select bands related to seizure outcome. An automatic detector using amplitude-duration-number thresholds was used. Interictal electrocorticography data sets of 8 min for each patient were selected. In the first training data set of 20 patients, the automatic detector was optimized to best differentiate the seizure-free group from not-seizure-free-group based on ranks of resection percentages of activities detected using a genetic algorithm. The optimization was validated in a different data set of 19 patients. There were 16 (41%) seizure-free patients. The mean follow-up duration was 21 ± 11 mo (range, 13-44 mo). After validation, frequencies significantly related to seizure outcome were 5.8, 8.4-25, 30, 36, 52, and 75 among low-frequency activities and 108 and 800 Hz among high-frequency activities. Resection for 5.8, 8.4-25, 108, and 800 Hz activities consistently improved seizure outcome. Resection effects of 17-36, 52, and 75 Hz activities on seizure outcome were variable according to thresholds. We developed and validated an automated detector for monitoring interictal pathological and inhibitory/physiological activities in neocortical epilepsy using a data-driven approach through outcome-guided machine learning. NEW & NOTEWORTHY Outcome-guided machine learning based on seizure outcome was used to improve detections for interictal electrocorticographic low- and high-frequency activities. This method resulted in better separation of seizure outcome groups than others reported in the literature. The automatic detector can be trained without human intervention and no prior information. It is based only on objective seizure outcome data without relying on an expert's manual annotations. Using the method, we could find and characterize pathological and inhibitory activities. |