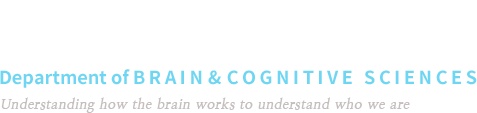
Faculty


Faculty Publications
Deep learning based low-cost high-accuracy diagnostic framework for dementia using comprehensive neuropsychological assessment profiles | |
---|---|
Year of publication | 2018 |
Title of paper | Deep learning based low-cost high-accuracy diagnostic framework for dementia using comprehensive neuropsychological assessment profiles |
Author | Hyun-Soo Choi, Jin Yeong Choe, Hanjoo Kim, Ji Won Han, Yeon Kyung Chi, Kayoung Kim, Jongwoo Hong, Taehyun Kim, Tae Hui Kim, Sungroh Yoon & Ki Woong Kim |
Publication in journal | BMC Geriatrics |
Status of publication | accepted |
Vol | 18 |
Link | https://bmcgeriatr.biomedcentral.com/articles/10.1186/s12877-018-0915-… 184회 연결 |
"Background The conventional scores of the neuropsychological batteries are not fully optimized for diagnosing dementia despite their variety and abundance of information. To achieve low-cost high-accuracy diagnose performance for dementia using a neuropsychological battery, a novel framework is proposed using the response profiles of 2666 cognitively normal elderly individuals and 435 dementia patients who have participated in the Korean Longitudinal Study on Cognitive Aging and Dementia (KLOSCAD). Methods The key idea of the proposed framework is to propose a cost-effective and precise two-stage classification procedure that employed Mini Mental Status Examination (MMSE) as a screening test and the KLOSCAD Neuropsychological Assessment Battery as a diagnostic test using deep learning. In addition, an evaluation procedure of redundant variables is introduced to prevent performance degradation. A missing data imputation method is also presented to increase the robustness by recovering information loss. The proposed deep neural networks (DNNs) architecture for the classification is validated through rigorous evaluation in comparison with various classifiers. Results The k-nearest-neighbor imputation has been induced according to the proposed framework, and the proposed DNNs for two stage classification show the best accuracy compared to the other classifiers. Also, 49 redundant variables were removed, which improved diagnostic performance and suggested the potential of simplifying the assessment. Using this two-stage framework, we could get 8.06% higher diagnostic accuracy of dementia than MMSE alone and 64.13% less cost than KLOSCAD-N alone. Conclusion The proposed framework could be applied to general dementia early detection programs to improve robustness, preciseness, and cost-effectiveness." |